Diagnose Engine Issues Precisely Before You Put Your Plane in the Shop
Operate More Economically
Extend Your Engine’s Reliability and Longevity
Your Engine is Talking –
But Are You Listening?
Your aircraft’s digital engine monitor and borescope images tell an important story about your engine’s health, but if you don’t know how to interpret the data, you may be unaware of developing mechanical issues or poor operating technique.
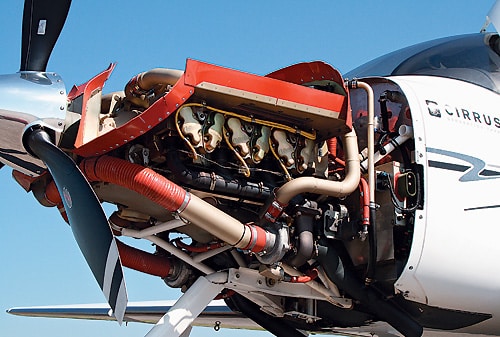
With SavvyAnalysis, you benefit from advanced engine reports…
Report Cards
The Savvy Platform simplifies vast amounts of data into easy-to-grasp infographics. Quickly distinguish between normal and abnormal readings and gain insights into how to address any issue.
Our Report Cards compare your aircraft’s critical parameters with all of the other aircraft of the same make and model that we track, allowing you to see how your airplane stacks up in comparison and determine any areas in which yours is an outlier.
Trend Reports
Our Trend Reports analyze key engine data parameters over time to identify statistically significant trends, such as increasing CHT or decreasing oil pressure, that you need to be aware of.
This timely information empowers you to proactively address any developing issues early, ensuring your aircraft’s powerplant’s continued health, performance, and efficiency.
FEVA™ Reports
Our FEVA™ (Failing Exhaust Valve Analytics) Reports safeguard your engine’s exhaust valves. FEVA combines a sophisticated system with machine learning technology to calculate a risk score for each of your engine’s cylinders using engine monitor and flight history data. If above-average risk is identified, we recommend a borescope inspection of those cylinders as soon as possible.
Since its inception in 2014, FEVA has analyzed over 3,000,000 GA flights, averting exhaust valve failures on over 60 occasions.
With the added edge of expert human analysis
Timely Data Interpretation & Pinpoint Diagnosis
At your request, one our our experienced GA Maintenance Analysts will examine your engine monitor data and borescope images for any indications that the engine is not operating normally. You’ll receive a report of the findings, including the likely causes of the problem identified. Our diagnostic report empowers you to tell your mechanic precisely what is wrong with your engine.
Wondering, “So what should I do about it?“ SavvyQA adds our expert A&P/IAs to your team to help you treat the problem efficiently and effectively.
Trend Recognition
Our experienced analysts can recognize distinctive data patterns associated with common engine problems like exhaust valve failures, ignition system issues, fuel system irregularities, and induction leaks, aiding in quick identification.
Better Powerplant Management
Our analysts’ expertise shared in each report helps pilots improve their powerplant management technique by uncovering anomalies caused by misunderstandings about correct operating procedures.
Optimal Maintenance
Our experts analyze your aircraft’s real-time combustion data to enable early detection of engine-related operating problems.
Identifying issues before the aircraft enters the shop allows for timely maintenance. It avoids costly, time-consuming “exploratory surgery” that may lead to maintenance-induced failures (MIFs) and diagnosis by guesswork (“shotgunning”) where the mechanic replaces various components and hopes for the best.
Cost Savings
By addressing issues proactively and avoiding unnecessary maintenance, SavvyAnalysis can result in significant cost savings for aircraft owners and operators.
GETTING STARTED IS SIMPLE…
Enroll in SavvyAnalysis
Click here to sign up for your account and pay a small fixed annual fee for immediate support:
Piston single: $189/year
Piston twin: $289/year
Sign In here and Upload Your Flight Data
Request an analysis of your engine data or borescope images by going to your Savvy dashboard and opening an analysis ticket. Provide any information you think would be helpful to our analysts.
We turn your raw engine data into actionable information to monitor your engine health, improve your operating techniques, protect the value of your piston, and save you money.
Receive An Easy-to-grasp Report
SavvyAnalysis FAQs
DO NOT EDIT
Is SavvyBasics or SavvyAnalysis right for me?
SavvyBasics provides 24/7 breakdown assistance and delivers Report Cards, Trend Reports, and FEVA alerts, providing sophisticated automated analysis of your aircraft’s data. However, it does not include human analysis by our team of experts.
SavvyAnalysis takes you two steps further by adding the expertise of our professional human analysts, who thoroughly examine your data, providing you with a deeper level of expertise, insight, and recommendations. Additionally, SavvyAnalysis extends its expert human analysis to your borescope images, ensuring a comprehensive assessment of your aircraft’s health.
What is a Digital Engine Monitor?
Digital engine monitors offer valuable insights into the engine’s combustion process, helping diagnose issues related to fuel, ignition, induction, exhaust, and turbocharging systems. For example, they can detect specific patterns associated with common problems like exhaust valve failures, ignition system issues, fuel system irregularities, and induction leaks. Additionally, these monitors can identify potential malfunctions within the monitoring system itself, such as failing temperature probes or wiring problems.
What engine monitor models are supported?
Virtually all of them, both certified and experimental. In the event we come across one that we do not support, we make it our first priority to add support.
Here is a partial list of the engine monitors that are presently supported:
- Advanced Flight Systems (AF-series)
- Avidyne (R8, R9, EX5000)
- Chelton EFIS
- Electronics International (UBG-16, MVP-50, CGR-30P)
- Dynon (all models including D10/D100-series, SkyView, HDX)
- Garmin (all models including G-1000, Cirrus Perspective, G3X, GI-275 EIS)
- GRT Avionics
- Insight Avionics (GEM 610, GEMINI 1200, G-series)
- J.P. Instruments (all 700-, 800-, and 900-series)
- MGL Avionics
- Ultra-FEI/Flightline AuRACLE
What is FEVA?
Failing Exhaust Valve Analytics (FEVA™) is a service included in every Savvy Basics, SavvyAnalysis, SavvyQA and SavvyMx subscription at no additional cost. FEVA uses your engine monitor data to predict the probability of a failing exhaust valve by combining two technologies. FEVA1 is an expert system that scans every flight you upload to the Savvy Platform i for the unique signature of a common exhaust-valve failure mode. FEVA2, significantly improves the accuracy of FEVA by employing machine learning technology based on data from over 5 million flights uploaded to the Savvy Platform. We provide you a “risk of failure” assessment for each of your engine’s cylinders. SavvyAnalysis Free clients can obtain the FEVA™ service by upgrading to the SavvyBasics, SavvyAnalysis, SavvyQA or SavvyMx subscription plans. Learn more about FEVA >>
What is a Savvy Report Card?
The Savvy Report Card is an infographic emailed to SavvyBasics, SavvyAnalysis, SavvyQA and SavvyMx clients on a regular basis. It contains graphical visualizations of your engine’s performance, including the values of important operating parameters during recent flights, and compares them to the performance of other aircraft of your same make and model that we track. Operational and maintenance recommendations are provided. The Report Card is produced entirely by our proprietary analytical software, and is based on the data you and others have uploaded.
The Report Card uses a “thermometer” graphic to depict your data and compare it to your aircraft’s cohort.
What is a Savvy Trend Report?
The Savvy Trend Report is an infographic emailed to SavvyBasics, SavvyAnalysis, SavvyQA, and SavvyMx clients on a regular basis. Like the Report Card, it contains graphical visualizations of your engine’s performance, but while the Report Card shows summaries of your data, the Trend Report shows individual data points from each of your flights included in the analysis, and identifies trends that are statistically significant. The Trend Report is produced entirely by our proprietary analytical software and is based on the data you and others have uploaded. The Trend Report uses a scatter diagram to depict your data and compare it to your aircraft’s cohort.
How do I get my Report Cards, Trend Reports and FEVA?
We provide Report Cards, Trend Reports and FEVA to you in two ways:
- By email. We send you updated reports when you have uploaded 12 new flights to our platform. If you haven’t received a Report Card in the previous 90 days, we send you one regardless.
- Online from your Savvy Aviation dashboard. You can provide the date range for the flights to include in your report.
What is Savvy's Borescope Initiative?
What are Flight Test Profiles and what kind of flights should I have analyzed?
Our experience has shown that reviewing data from “day to day” flights usually doesn’t provide much that is diagnostically meaningful.
We recommend flying the Flight Test Profiles and requesting analysis just prior to the annual inspection or other significant scheduled maintenance, and then again after the maintenance is complete.
Of course, if there is an in-flight anomaly/event that causes you concern, we encourage you to request analysis of the flight. Even in those cases, we encourage our clients to perform the Flight Test Profiles if it’s possible to do so safely, as they give us the best chance to pinpoint the issue.
I am a SavvyMX or SavvyQA client, do I need to enroll in SavvyAnalysis?
Can I upgrade from SavvyAnalysis to SavvyMX or SavvyQA?
Yes, you can! And if you do, you will receive a pro-rata credit for the unused portion of your SavvyAnalysis fee applied against your first year SavvyMx or SavvyQA subscription fee. Our software does this automatically when you upgrade.
Will your expert analysts review the data from all of my flights?
Will you talk to my mechanic about troubleshooting my engine’s problems?
To keep our service affordable we must limit our involvement to interpreting the data. We think we can provide the most value by focusing on your engine monitor and borescope data, and suggesting likely starting points for troubleshooting any problems we see. If you prefer us to be more involved in your maintenance, consider enrolling in our SavvyMx maintenance-management plan or SavvyQA consulting plan. Both of these programs include full access to SavvyAnalysis at no additional cost.
With SavvyAnalysis, you understand what the data means, but do you find yourself asking, “So, what should I do about it?”
Perhaps you should consider SavvyQA:
SavvyQA includes all the services of SavvyAnalysis plus unlimited maintenance and operations consulting.
Manage your maintenance with our team of trusted technical experts in your corner. Whenever you have maintenance questions, our unrivaled team of maintenance experts has the answers!
Savvy Offers Four Annual Plans
Choose the Plan that’s Right for You
BEST VALUE
Monitor Your
Engine's Health
SavvyBasics
$99/yr
Access to engine data graphing platform
Unlimited uploads of engine monitor data
and borescope images
Breakdown assistance away from home 24/7/365
FEVA (failing exhaust valve analytics) reports
Report Cards + Trend Reports
More About
SavvyBasics >
BEST VALUE
Experienced Analyst's
Interpretation of Your Plane's Data
SavvyAnalysis
$189/yr
Access to engine data graphing platform
Unlimited uploads of engine monitor data
and borescope images
Breakdown assistance away from home 24/7/365
FEVA (failing exhaust valve analytics) reports
Report Cards + Trend Reports
Expert analysis of your engine data and borescope images
More About
SavvyAnalysis >
BEST VALUE
Expert A&P/IA Advice
When You Need It
SavvyQA
$449/yr
Access to engine data graphing platform
Unlimited uploads of engine monitor data
and borescope images
Breakdown assistance away from home 24/7/365
FEVA (failing exhaust valve analytics) reports
Report Cards + Trend Reports
Expert analysis of your engine data and borescope images
Expert maintenance consulting with our A&P/IAs
Expert second opinions
Shop and supplier recommendations
Parts research and sourcing
Review of work orders and invoices
More About
SavvyQA >
BEST VALUE
Full-Service
Maintenance Management
SavvyMx
$899/yr
Access to engine data graphing platform
Unlimited uploads of engine monitor data
and borescope images
Breakdown assistance away from home 24/7/365
FEVA (failing exhaust valve analytics) reports
Report Cards + Trend Reports
Expert analysis of your engine data and borescope images
Expert maintenance consulting with our A&P/IAs
Expert second opinions
Shop and supplier recommendations
Parts research and sourcing
Review of work orders and invoices
Comprehensive maintenance management
Prebuy management nationwide (for buyers)
Dedicated A&P/IA account manager
We work directly with your shop and mechanics
More About
SavvyMX >
NOT READY TO ENROLL IN A PAID PLAN?
Try SavvyFree
Savvy clients at every level have access to The Savvy Platform – The world’s leading software for analyzing information gathered from piston aircraft data monitors.
Analyze your engine data for free using the same powerful flight data charting tools our professional data analysts use with SavvyFree.
JOIN THE 10,000+ GA OWNERS USING THE SAVVY PLATFORM
Gain access with every Savvy Plan from SavvyFree to SavvyMX
The Savvy Platform transforms your data into easy-to-understand infographics. Upload, store, and view engine monitor data in graphical form to aid your analysis.
Drag and drop your borescope images in The Savvy Platform, tag them by cylinder view, annotate them to indicate condition, then generate PDF reports that combine your images and annotations in an elegant report format.
Never worry about losing essential flight metrics with unlimited uploads of engine monitor data. Every flight you upload has its data automatically scanned by our proprietary algorithm.
The user interface for The Savvy Platform is web-based, so it works with PCs, Macs, tablets, and mobile devices. You can access SavvyAnalysis from almost any device with Internet connectivity and a standard browser (Chrome, Safari, Firefox, Edge, etc.)